11.03.21
By Paul Manberg
How do we get more value out of limited health care dollars? One strategy is to use a risk stratification tool that helps avoid expensive downstream events by identifying which patients should be selected for additional surveillance and earlier intervention if needed. AI-driven predictors that use administrative data are attractive because the data is readily available and adheres to a common format. But does the predictor retain its power when regional coding differences are considered? In the case of one predictor the answer is yes.
RSI, an all-cause mortality predictor, has once again been shown to be a reliable baseline risk indicator. In a recent paper published in BMJ Open, the study authors concluded that “The RSI reliably tracked mortality, independently of regional coding practices and thus represents a promising method for adjusting baseline patient risk and comparing hospitals across various regions of the USA.” 1
Researchers from Health Data Analytics Institute collaborated with a team from the Lown Institute, the Harvard T.H. Chan School of Public Health and Outcomes Research at the Cleveland Clinic to describe how RSI can be used to account for regional variations in underlying health status and coding practices. According to the Cleveland Clinic’s Outcomes Research site: “The Risk Stratification Index (RSI) is an open source, nationally validated, risk stratification methodology that permits outcomes such as duration of hospitalization and mortality to be compared equally across institutions.”
The objective of this study was to test the hypothesis that regional variations in diagnostic frequency in inpatient records are a reliable indicator of true underlying health risks. Two methods of risk stratification were compared: (1) the number of chronic conditions; and (2) quartiles of RSI-defined risk. In addition, six socioeconomic indicators (percentages of smoking, obesity, education more than college, African/Black-American status, family household marriage status and median income) were also studied as potential factors associated with the primary all-cause annual mortality outcome measure.
Results demonstrate that regional diagnostic frequencies among inpatients are not a consequence of over-coding but do reflect higher baseline health risk. In addition, findings also indicate that RSI accounts for the impact of common socioeconomic determinants of health and offered greater resolution than counts of chronic conditions. The full paper is available here
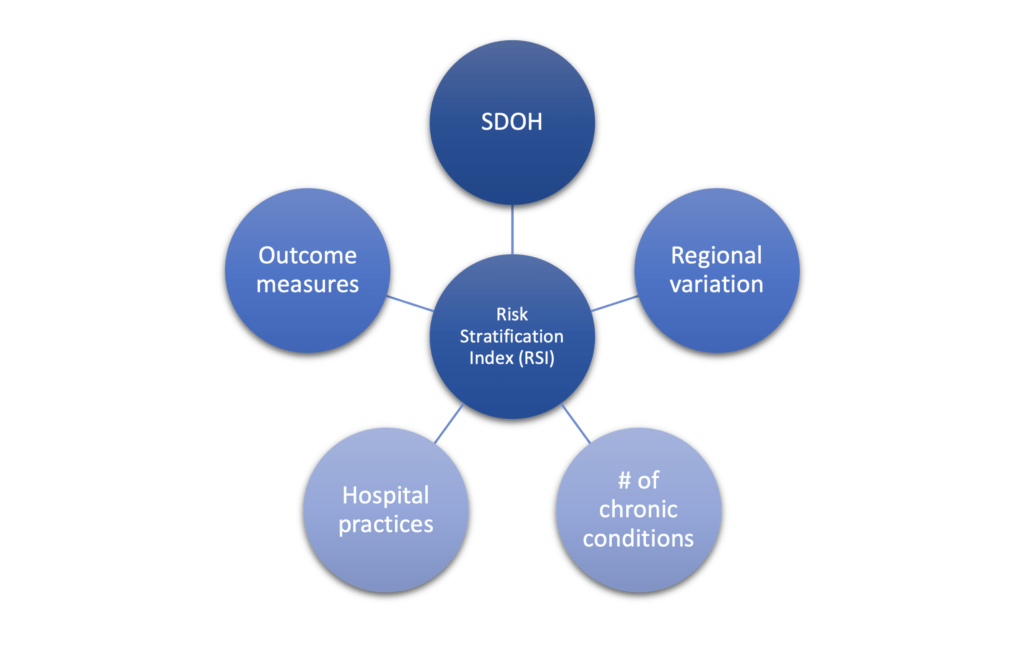
RSI accounts for multiple inputs: including socioeconomic factors, regional health status variation, the number of chronic conditions, hospital practices, and outcome measures
Study authors and affiliations
Linyan Li: School of Data Science, City University of Hong Kong, Hong Kong, China and the Department of Environmental Health, Harvard T.H. Chan School of Public Health, Boston, MA, USA
George F Chamoun: Health Data Analytics Institute, Dedham, MA, USA
Nassib G Chamoun: Health Data Analytics Institute, Dedham, MA, USA
Daniel Sessler: Outcomes Research, Cleveland Clinic, Cleveland, OH, USA
Valérie Gopinath: Lown Institute, Needham, MA, USA
Vikas Saini: Lown Institute, Needham, MA, USA
SHARE